Approaches to AI Accessibility Aids for Publishing Professionals
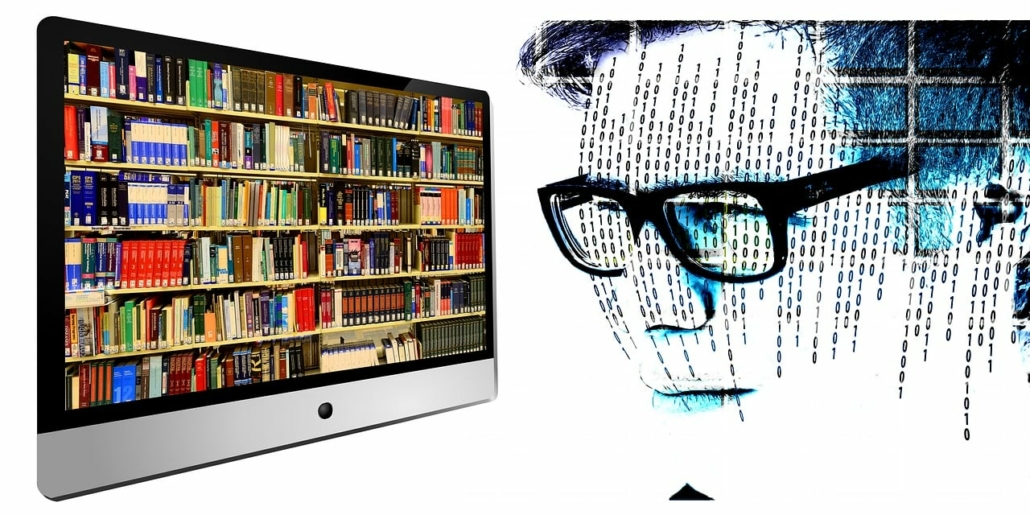
As countries around the world introduce accessibility legislation that more explicitly includes digital publications, more and more publishers are finally getting serious about making accessibility happen. Discussions are beginning to move from a focus on corporate responsibility and ethical framings toward more economically straightforward terms of compliance and risk. And this is all good news!
Simon Mellins, accessibility and publishing expert, looks at some of the possible approaches to AI being made by the publishing industry.
But as these conversations develop it is natural that there is a call for the types of resources that can tackle some of the key hurdles, and companies are understandably looking for efficiencies and shortcuts (not necessarily a dirty word!), especially when it comes to tackling often enormous backlists.
In particular, anyone in a board room or at the London Book Fair this year will have almost inevitably been asked (or pitched to) about using AI to solve some of these challenges, particularly when it comes to transformation at scale. This article gives an overview of where AI might fit into accessible content creation and remediation, but also carries some strong notes of caution and care. I’ll avoid mentioning specific products and services, and instead talk about the general landscape and what to be aware of.
Markup Madness
Can AI help us make the coding of our publications more accessible? There certainly are services out there offering to remediate website code, and EPUB is mainly comprised of web code. But can it reliably make the right decisions?
Just as with the web, making accessible book content fundamentally comes down to 2 core concepts: Structure and semantics.
Structure refers to things like the logical ordering of elements (e.g. not putting an H2 before an H1), correct use of HTML (e.g. don’t just make everything a <div>) and logical navigation. Whilst AIs can take a good stab at this, many of these decisions are subtly subjective, and it’s going to be very difficult for anyone non-human to impute the exact meaning and purpose of every element of a publication.
In a publishing context, really this just comes down to good inputs (well-structured DocX or other formats), correctly marked up layout (usually InDesign) files, and/or well-designed specifications and templates for your chosen vendor to use. Many vendors are pitching AI more at remediating older files, but the same problems of subjectivity are there, and deputising a vendor – let alone an AI – necessarily means giving up a lot of control and choice. And all this assumes a markup assistant is actually tuned for EPUB, where considerations are subtly different to those of the web.
Semantics is a linked concept, insofar as everything in the publication needs to be intentional as well as clearly and correctly labelled. This is enhanced with the use of semantic tagging (i.e. epub:type and ARIA) to clearly identify parts of a publication, section, page etc. These tags can also be used by Assistive Technologies such as screen readers to provide deep navigation and other functionality. In EPUB we refer to this as structural semantics, i.e. giving meaning to a structure. This is a great term, which I group together with image description (more on that later) as ‘semantic enrichment’. It may seem confusing that I listed structure and semantics separately but then dropped this term, but the key is that all of this is linked. As ever with accessibility, the key is not to try to presume or guess every possible need, but instead to make everything as explicit and logical as possible so that any given assistive technology has the right ‘hooks’ to present the content to the user however they need it.
Semantics are a really hard problem for AI, but in principle a solvable one. Many web-focused coding assistants have been launched. AI (or rather Machine Learning in this context) is strongest when detecting and repeating patterns, and published works are often relatively predictable and formulaic, so there could be a place for AI here in remediation – i.e. fixing up old, inaccessible files. For authoring accessible files in the first place, however, if you’re even considering these tools, something has gone wrong with either your workflow or your specs and templates. If those are done right, with good inputs and careful control and QA, the resulting files will already be accessible, as well as far easier to transform into any future format that you need.
Finally on this, remember that bad semantics are often worse than no semantics at all. Assistive Technology users are sadly all too used to having to find their own ways around inaccessible digital publications, but ones with sloppily applied semantics may actually be more unpredictable and obstructive than ones where they’re missing altogether. So trusting an AI to get all this right carries substantial risk.
Image Description
Ok ok, this is probably the part you clicked on this article for. I know – describing every image you publish, especially for the backlist, feels almost completely impossible. But the European Accessibility Act (EAA) is pretty clear that backlist is included, and states that have implemented the directive into their domestic laws have taken that interpretation so far (albeit some with generous grace periods), so the debate is hardly relevant anymore. So the idea of using automation to speed up and potentially reduce costs for this process is a very logical fit for AI, and many vendors are launching products to attempt this. But there are some major caution points that a vendor is unlikely to want to focus on too much.
Hosting and IP
Firstly, when assessing any AI solution for any part of your workflow, pay close attention to who is hosting the AI. Most AIs are built to learn from every interaction they have, and you don’t want your copyrighted materials and other IP used for that. This form of ‘copyright erosion’, as an intellectual work loses its relationship to its source and becomes part of ‘general knowledge’, is a massive slippery slope problem and a huge legal risk.
There’s also the blunter risk of an unscrupulous AI firm outright-copying your material once you’ve fed it to them. Thus any AI solution needs to be in an isolated/sandboxed server instance (real or virtual) with a company you trust, and with contractual assurances of its safety and that nothing you upload will be retained or used for training a wider model outside of your own instance.
Choosing Images
Remember that not all images require description. Somebody still needs to go through the work and establish which images are decorative, which are described sufficiently by their caption or by nearby text, and which for any other reason should not have image descriptions. As with semantic enrichment, bad or unnecessary image descriptions are often worse than no image descriptions at all, and repetition in particular will really irritate your assistive technology users. Additionally, you’ll be paying to describe images needlessly.
Workflow
Having a capable AI is one thing, but how do the images get sent to the AI system, and how are the generated image descriptions integrated back into the publication? Keep track of how much extra work is being created in all this, as opposed to generating the descriptions in other parts of your own internal process using authors or editorial staff.
Context is Key
Probably the biggest restriction of using AI for image description is context. The meaning of a given image in isolation is often very different to what that same image might mean in a specific context. For instance, a picture of someone in a boat holding up a fish would need more specific description in the context of a fishing book, such as the particular fish type, whereas in many other kinds of book this information might not be relevant. The full name of a person, or introducing each person in an image, may not be necessary depending on what’s come before in the publication. We are trying to create quality semantics here, not junk that screen reader users have to dredge through.
Indeed, context also means ensuring the descriptions all fit together nicely, avoiding unnecessary repetition of information, with consistent assumptions about audience knowledge (including knowledge accumulation through a publication). Most AI systems for image description simply cannot know all this – they aren’t ‘reading’ the whole publication and they don’t know your audience. Any vendor who claims their system can infer all this by ingesting the whole book would need to provide robust evidence of this (how well has the AI understood the work), as well as doubling-down on copyright safety assurances.
Editorial Voice
Whilst this remains a contentious topic, increasing consensus is building that image description is an editorial, rather than technical task. The Platonic ideal of completely ‘mechanical’ alt text that purely describes the image, with absolutely no value judgement or interpretation, is simply not a realistic model in the messy, contextual world of a published work. What if the AI makes a bad assumption, or says something offensive or otherwise not inline with your organisation or the author’s political values/’voice’?
In addition, remember the author. The practicalities of running every image description past them may be problematic, but the conversation at least needs to be had, particularly if your organisation has any code of conduct or moral right agreements that may be relevant. This is something to be discussed among editorial, production and legal stakeholders to decide on an appropriate approach.
When using humans for image description, organisations can guide decisions by creating an ‘Image Description Style Guide’, a document I usually suggest to and develop with clients, and that I recommend all organisations to organically develop over time as they bring their image description workflows to life. But integrating this with an AI is not straightforward and will often rely on a human QA component, at which point the time and cost savings must be questioned.
As with everything I’m saying here – this could change as the technology evolves, but these are the sorts of things about which to interrogate any new product or service in this space.
AI as a Starting Point
Many of the AI-based image description solutions hitting the market now are offering a hybrid approach, whereby a generative AI LLM creates a basic description and a human (ideally a Subject Matter Expert/’SME’) checks and edits it after the fact. They claim this is substantially faster than a human doing the whole thing, and assuming human time is more expensive than AI, they claim this works out cheaper.
Of course this doesn’t solve the problem of initial image selection/triage I covered earlier, nor does it fully incorporate context, unless that SME really knows the work intimately and not just the broad topic area. Indeed correcting or rewriting/replacing a useless AI description could take longer than writing one in the first place. And, of course, the images need to be extracted and the descriptions integrated into the work.
Additionally, the claims about time and therefore cost savings are just that – claims – with no controlled trials likely to be carried out to confirm them, so whilst you can take these companies’ word for it, really there’s nothing better than doing your own testing and comparisons.
Wrapping Up
As you’ll have gathered by now, there is no single answer to whether AI can help with this challenge. But it is crucially important for publishing professionals to know the risks and realities, and for vendors to know the concerns publishers have and to build their offerings around them.
The time to get working on a frontlist accessibility workflow is now. As for backlist, the chances of every publisher having every backlist title ready for the EAA deadline of 28 June 2025 are next to nil – but one thing that I believe will prove vitally important is having a planned and responsive remediation system ready-to-go. That is why these questions need to be resolved now, and a workflow needs to be developed. This might involve deep organisational change, including a resummation of what it means to be an ‘editor’ in an age when content is ‘data’ and not just printed works. But perhaps that’s for another article!
AI for accessibility in publishing is an ongoing conversation, and the right approach varies depending on your setup and content. Feel free to reach out to me directly via my website, and please do join the Publishing Accessibility Action Group over at paag.uk and on LinkedIn to stay up to date on the latest developments in everything accessibility. You can also check out my upcoming podcast where we’ll be talking about accessibility, AI and lots more.
This article was kindly submitted to Inclusive Publishing by Simon Mellins, Digital Publishing Consultant (simonmellins.com)